Johns Hopkins researchers have developed a powerful new AI tool called Splam that can identify where splicing occurs in genes—an advance that could help scientists analyze genetic data with greater accuracy, offering new insights into how genes function and mutations contribute to disease.
Their results appear in Genome Biology.
Key Takeaways
- An AI tool called Splam analyzes genetic data with greater accuracy than existing methods to explore potential links between mutations and disease
- Splam works by recognizing splice sites, where cells trim away the non-essential portions
- Recognizing splice sites is a crucial step in assembling gene transcripts to identify the functional parts of DNA and the roles they play in the body
"Precisely identifying splicing sites is key to understanding how cells interpret genetic instructions," says co-lead author Kuan-Hao Chao, a doctoral student in the Whiting School of Engineering's Department of Computer Science who is affiliated with the Center for Computational Biology (CCB). "Splam lets us analyze genetic data with accuracy and efficiency, showing how mutations affect our health and why the same gene can produce different proteins in different conditions."
He is joined on the project by his advisors—Steven Salzberg, the Bloomberg Distinguished Professor of Computational Biology and Genomics and the director of the CCB, and Mihaela Pertea, an associate professor of biomedical engineering and genetic medicine with a secondary appointment in the Department of Computer Science—as well as Alan Mao, a fourth-year undergraduate double majoring in biomedical engineering and computer science.
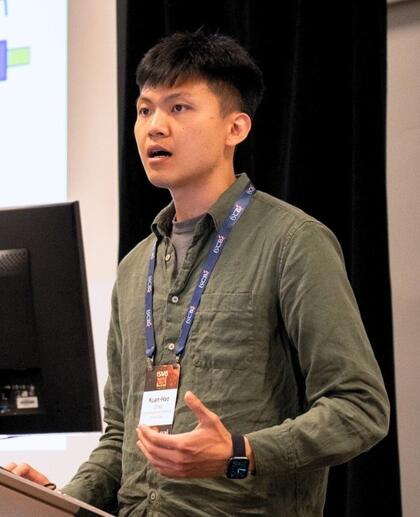
Image caption: Kuan-Hao Chao
Image credit: Whiting School of Engineering
Cells rely on genes to guide their functions, with each gene containing both useful instructions (called exons) and non-essential segments (called introns). Splicing is the process by which cells trim away the non-essential portions, retaining only what is needed.
According to the researchers, recognizing splice sites computationally is a crucial step in accurately assembling gene transcripts in modern genetics studies, where RNA sequencing experiments measure the level at which a gene is expressed—basically, whether it's turned on or off—in different conditions.
"For example, cancer researchers often use RNA sequencing techniques to compare gene expression in healthy versus cancerous cells," says Chao.
Identifying splice sites is also important in annotating genomes, which involves identifying which parts of our DNA are functional and what roles they play in the body. One familiar application of genome annotation is in genetic testing services, such as those offered by companies like 23andMe. These tests analyze parts of your genome to tell you about your ancestry, health risks, and genetic traits. Genome annotation makes this possible by identifying and interpreting these regions of the human genome.
Compared to the state-of-the-art "SpliceAI" tool, the Hopkins team's "Splam" method uses a much shorter DNA sequence window to predict RNA splice sites, making its model more biologically realistic and feasible for use in research, Chao says.
The team's Splam algorithm takes a DNA sequence of 800 nucleotides—400 each of adenine (A), cytosine (C), guanine (G), and thymine (T) on both sides of potential donor and acceptor sites—and outputs the probability for every base pair being a donor site, an acceptor site, or neither.
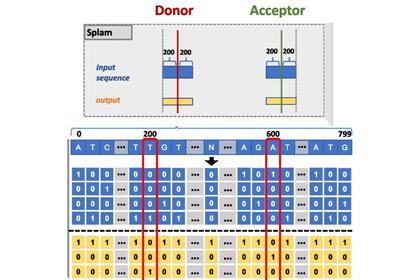
Image caption: Compared to the state-of-the-art “SpliceAI” tool, the Hopkins team's "Splam" method uses a much shorter DNA sequence window to predict RNA splice sites, making its model more biologically realistic and feasible for use in research
Image credit: Whiting School of Engineering
"Our algorithm attempts to recognize these donor/acceptor sites in pairs, just as a spliceosome 'molecular machine' does in the cell when it cuts out an intron," says Chao.
The researchers developed their algorithm to recognize splice junctions within a window of 800 nucleotides—a far smaller region than the 10,000 nucleotides required by Splice AI. The team reports that despite requiring less genomic data, Splam achieves better splice junction recognition accuracy than SpliceAI.
After training their deep learning model on human DNA, the researchers ran additional tests on other species' genetic codes.
"A frequent concern about deep learning methods is whether they simply memorize their training data or if their predictive models will work on data that diverges from what they have seen in training," Chao says. "So to evaluate whether Splam had learned more general splicing rules, we collected data from three successively more distant species and applied the algorithm to each of them without re-training."
The team chose the genomes of a chimpanzee, a mouse, and a flowering plant in the mustard family. Their subsequent experiments demonstrated that Splam's biologically inspired design still produced highly accurate results on these more distant DNA sequences—showing that their method had indeed learned essential splicing patterns shared across many animals and plants.
The team's next steps include applying its model to more species and integrating its method into existing RNA sequencing pipelines for practical use in transcriptome assembly.
"Our method has immediate applications in improving transcriptome assembly and reducing splicing noise, making it valuable for a wide range of genomic studies," says Chao. "We hope that Splam will contribute to the better understanding of our genomes and the genes within them."
Posted in Health, Science+Technology