With a three-year grant from the Department of Energy, a team of researchers from Johns Hopkins and partner organizations are launching the Synthesis and Processing Informed by Rational Algorithmic Learning Center, or SPIRAL, a multi-institution effort to harness the power of artificial intelligence to accelerate the optimization of low-cost materials for solar energy.
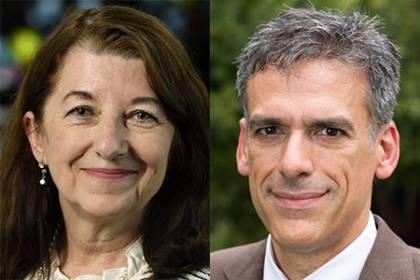
Image caption: Paulette Clancy and Rigoberto Hernandez
Johns Hopkins faculty members Paulette Clancy, head of the Whiting School of Engineering's Department of Chemical and Biomolecular Engineering, and Rigoberto Hernandez, professor of chemistry in the Krieger School of Arts and Sciences, will help lead the project's machine learning component.
SPIRAL is one of only 10 projects to receive support through a DOE initiative focused on leveraging cutting-edge research tools for the development of low-cost energy technologies. The SPIRAL center includes leading scholars from Cornell University, the University of Virginia, the National Renewable Energy Laboratory, the Pacific Northwest National Laboratory, and the Lawrence Livermore National Laboratory, a group that Clancy describes as a "dream team of experimental and computational researchers."
SPIRAL draws upon the JHU team's expertise in metal halide perovskites, a promising, high-performing class of materials for solar cells that offer unique advantages, including that they are made from earth-abundant materials in solution at ambient temperature and are compatible with low-cost application processes, such as inkjet printing and slot-die coating.
To realize the promise of this class of materials, the research team must not only understand what composition of metal halide perovskites is most effective, but also how to optimize the processing of them.
"While the potential benefits of the materials are tremendous, solving these fabrication problems involve far more variables than can be studied in an Edisonian trial-and-error manner," Clancy says. "This is an ideal situation in which machine learning can help tame an otherwise overwhelming number of options."
The Hopkins group is building on its recent successes in the characterization and prediction of multiscale properties of materials using design and control of properties at the molecular to middle scales.
"We are excited to leverage our experience in machine learning models to characterize sustainable nanoparticles to address the challenge to optimize formulations for a new class of energetic materials," says Hernandez, noting that the team's experimental members will benchmark the models and provide the database for making new models. "Information will flow in both directions between teams, and indeed can be considered as spirals, going clockwise and counter-clockwise in parallel, leading to a seamless integration to converge into new novel materials."
Steve Binkley, the acting director of the DOE's Office of Science, says the work has the potential "to accelerate the discoveries needed for more efficient energy generation, storage, and use, while eliminating or reducing the emission of greenhouse gases and the use of critical resources."
Posted in Science+Technology
Tagged chemistry, materials science, sustainability, artificial intelligence, machine learning, solar power