The polls got it wrong.
That was one of the immediate takeaways on election night, when Republican Donald Trump—the consensus underdog in the presidential race according to nearly all major public opinion polls and predictions—scored a surprise victory against Democrat Hillary Clinton.
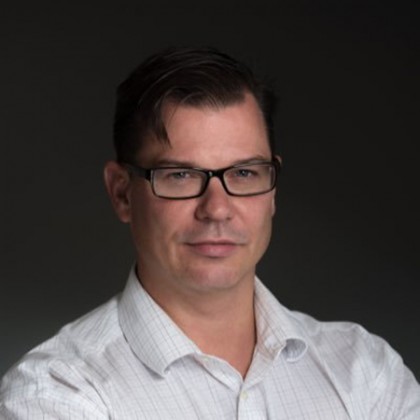
Image caption: Clifford Young
Though Clinton will win the popular vote—her lead is more than 2.3 million and growing with votes still being counted—the nuances of the Electoral College gave Trump a victory that few forecasters saw coming. That miss has led to new scrutiny of pollsters and prognosticators, whose data-driven work has taken on an increasingly prominent role in political coverage in recent years as a complement to traditional "horse-race" reporting.
Clifford Young is a professorial lecturer at the Johns Hopkins University School of Advanced International Studies whose research specialties include public opinion trends and election polling. He also leads global election and political polling risk practice at Ipsos, a market research firm that published polling data throughout the 2016 election cycle.
The final Ipsos forecast, published a day before the election, gave Clinton a five-point national lead (44% to 39%) among likely voters and a 90% chance of winning the election.
Young spoke to the Hub about where the Ipsos poll (and many others) went wrong, how the instruments can improve, and what pollsters can do to rebuild public trust.
Why did the polls get this election wrong?
There are multiple ways to forecast elections. One way is to have long-term forecasting models, where typically you aggregate a bunch of elections and use that to come up with who has a greater chance of winning. Another way to do it is much more close in, and that's polling. And so basically, this entire election cycle, the long-term forecasting models suggested a Republican victory, and the polling, for the most part, suggested a slight Clinton lead.
It's a difficult place to be because you have different pieces of important evidence that had contradictory stories. And so ultimately, what happens is, when you're far out from an election, you trust your long-term forecasting models more, and when you get closer to an election, you trust your polling more.
What I would say is the following: The long-term forecasting models, I think, were spot-on. More importantly, they suggested a very uncertain election. I think the biggest place that we made a mistake—and not just pollsters but data journalists and forecasters as well—is in our level of conviction about a Clinton victory. I think overall, as an industry, we should have been much more uncertain about the outcome.
But ultimately what happened with the polling, at the national level the average of all the polling gave Clinton a lead of three [percent]. When all the votes are counted, we're probably going to be at 1.5 percent. Not to defend polling, but at the national level, for the most part, we picked the winner of the popular vote. Where the polling fell down was in the swing states—more specifically, in Pennsylvania, Michigan, and Wisconsin, which ultimately went Trump. So it altered the evaluation a little bit.
I think we did a reasonable job of saying who is going to win the popular vote. We did not do a good job of saying who is going to show up on election day in each of the states.
The biggest challenge of pollsters is not so much doing a robust poll that's representative of the population, but actually predicting who will show up on election day. On the margins, that's where we failed. We failed to show that it was going to be lower turnout in some places, a differential turnout—that is, more Republicans than Democrats—in some of the key states like in Pennsylvania, where some Democrats stayed home.
Is there a way to improve individual polls to better predict things like turnout?
Where we are at now with the science of polling is, we do a good job of having a representative poll of the population. We have pretty good methods that can rank-order people in terms of their relative propensity to show up on election day. So I can tell you, if it's a higher turnout election, it probably looks more Democrat. If it's a lower turnout election, it probably looks more Republican.
Where we have a serious blind spot is actually telling you whether it's going to be a higher turnout or a lower turnout election. The big place for research and development, in my opinion looking forward, is in developing much more robust predictive models in terms of turnout: is it going to be a high turnout election, a low turnout election, an average turnout election, a differential turnout, where more of one side shows up than the other side? That's where we need to focus.
Obviously understanding where millennials versus older people, how much they show up, is important, and those are the sorts of inputs that would go into a model. But overall, conceptually speaking, that's sort of where we need to focus.
Do you think the improvements you mentioned will be enough to restore the public's faith in polls?
If we're super honest with ourselves, the question is, are we doing a disservice by selling a false bill of goods when it comes to precision. The biggest problem is that the industry as a whole said that Clinton was probably going to win, and actually Trump won. And so, picking the wrong winner, even if it's within a point or two—I mean really, we're talking about 150,000 votes in those three states. Less than 150,000 votes. It was razor thin in those three big states that went Trump—Pennsylvania, Wisconsin, and Michigan. But that said, the burden is on us to pick the right winner. Ultimately the question is how precise can our instrument ever be?
We're dealing with a future behavior, not a present behavior, and there can be last-minute decision changes, or people might even state certain things to a pollster but when they get into the election booth they might think something different—that's called strategic voting. I might intellectually be against Trump, but in the end I'm like, "You know what, four years of Trump's not going to kill us, I just might vote for him just to send a message." And so we have a hard time capturing that indecision. And let's be frank—this election was a game of inches. Again, the national polls will probably be off 1.5 points, and we're talking about 150,000 votes in these three key swing states. If it goes a little bit differently, we're not having this conversation.
Yes, we definitely need to invest in having better forecasting models for turnout. Definitely. And at Ipsos, we're investing heavily in that already. But I think we need to be honest with ourselves about the tools at hand, that perhaps they're not as precise as we've been communicating them to be. So when a Nate Silver, or a Sam Wang at Princeton, or one of these data journalists comes out and says, "There's a 99.9 percent chance of Clinton winning," are we being honest with ourselves? Can human behavior ever be that precise? We need to be more humble and a little bit more understanding in that there's a lot more imprecision than the narrative actually suggested that there was.
What other challenges do polls—and pollsters—face going forward?
Stepping back a bit, I believe from my understanding of the data at hand that we in the Western world are heading into a political super-cycle of uncertainty, where people believe that the system is broken, they're willing to take chances on anti-establishment candidates, and where our past behavior is not prologue. What we often do when we predict behavior is to use back behavior. And while there's some correlation there, it's attenuated a bit, and so this just kind of feeds into my initial point which is that we as an industry should have had much less conviction about what was going to happen and much greater appreciation for the vagaries of human behavior and understand that we are in a context that is much more unstable and more unpredictable, to be quite frank. And I think that looking forward, not only will we obviously invest in better instruments, but we have to have, as analysts and decision-makers, a greater appreciation for this uncertainty.
I think we're heading into a very populist time, where people are scared about the future, they don't see a path forward, and they're going to increasingly be likely to make decisions that are not linked to their past decisions. There's a supermajority belief in the United States that the system is broken, and that traditional parties and politicians no longer represent the average person, that the system is rigged. And so both the right and the left believe that here. So we had Bernie Sanders and we had Trump as two sides of the same coin in respect to this worldview. We just got done with a poll in 26 countries which says that it's universally the case, everywhere. People believe this, generally speaking.
And so the question is what are the macro-drivers of this overall trend. And it's globalization, it's automation, it's also a changing demographic—in both Europe and the United States, you have spikes in foreign-born populations, birth rates of low replacement, we have increasingly nonwhite populations. And so you have demographic and cultural changes, combined with declining disposable income and declining purchasing power, all driven by these very similar global macro-trends, and people are just uncertain about the future. So let's call that human uncertainty. That uncertainty of people's outlook translates into the behavior, and they're willing to make different sorts of choices—in this case political choices—than they have in the past. In our case, that makes our job much more difficult.
Posted in Voices+Opinion, Politics+Society
Tagged political science, election 2016, donald trump, polling