- Name
- Johns Hopkins Media Relations
- jhunews@jhu.edu
- Office phone
- 443-997-9009
The process of testing new solar cell technologies has traditionally been slow and costly, requiring multiple steps. Led by a fifth-year PhD student, a Johns Hopkins team has developed a machine learning method that promises to dramatically speed up this process, paving the way for more efficient and affordable renewable energy solutions.
"Our work shows that machine learning can streamline the solar cell testing process," said team leader Kevin Lee, who worked with fellow electrical and computer engineering graduate students Arlene Chiu, Yida Lin, Sreyas Chintapalli, and Serene Kamal, and undergraduate Eric Ji, on the project. "This not only saves time and resources but opens new possibilities for clean energy technology development."
The team's results appear in Advanced Intelligent Systems.
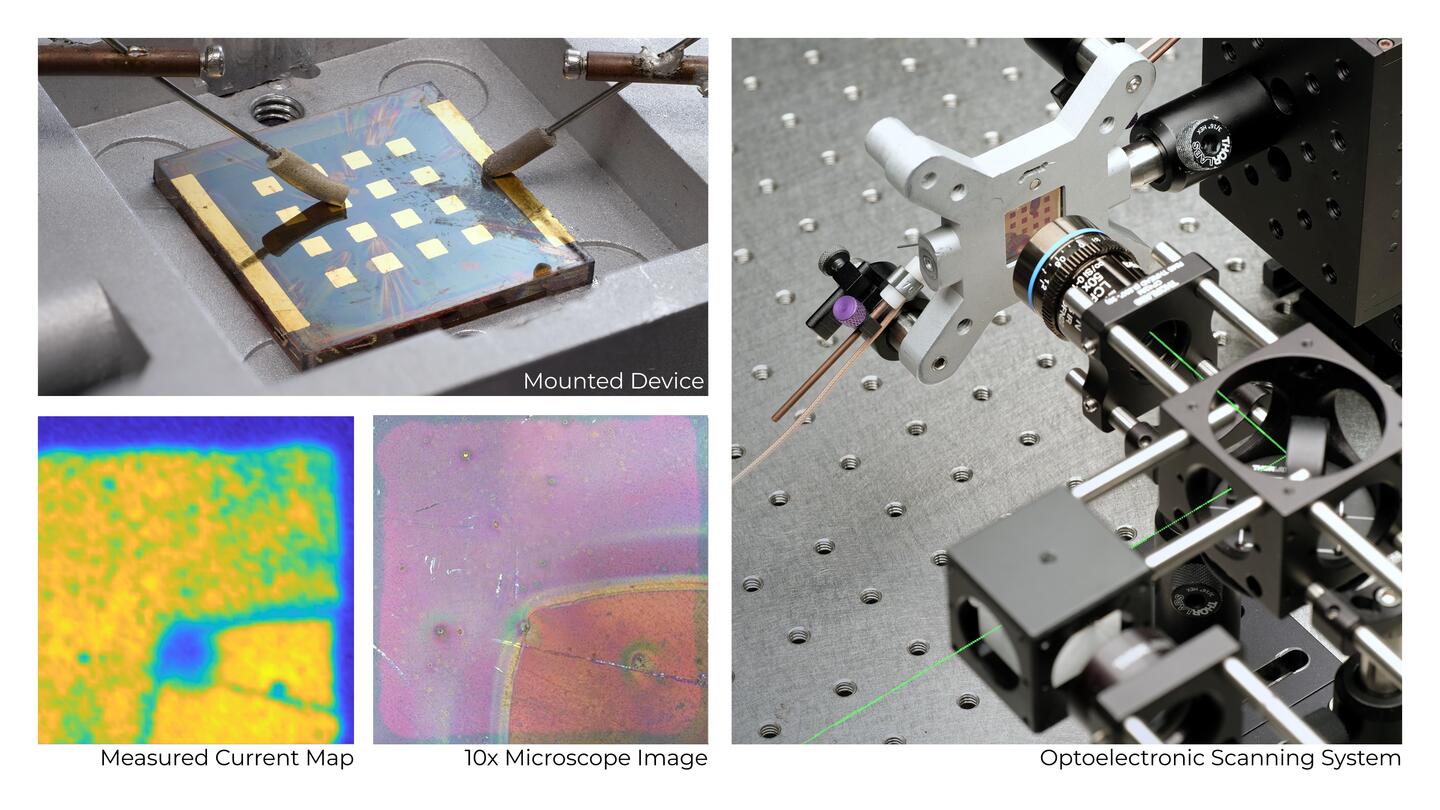
Image caption: In this new method, the scanning instrument (right) is used to measure current and optical maps (lower left) of solar cell arrays (top left).
A major hurdle in commercializing new solar materials and devices is the lengthy fabrication-testing-iteration cycle. Optimizing a new solar cell material for the market is an arduous process: After a device is made, multiple time-consuming measurements are needed to understand its material properties. This data is then used to adjust the fabrication process, repeating the cycle.
The new method drastically reduces this time by extracting all the materials' important characteristics from a single measurement. Unlike other methods trained on computer-simulated data—which often produce inaccurate results—the Hopkins team's approach uses real-world data. Their neural network collects thousands of data points from one solar cell, capturing complex properties and variations caused by defects, such as spin-casting streaks, cracks, and contaminants, and eliminating the need to fabricate thousands of solar cells.
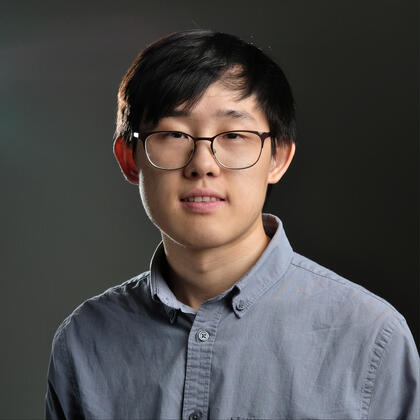
Image caption: Kevin Lee
"Kevin's method has the potential to speed up photovoltaic development times," said Lee's adviser and study co-author Susanna Thon, an associate professor of electrical and computer engineering at JHU's Whiting School of Engineering and associate director of the university's Ralph O'Connor Sustainable Energy Institute. "Instead of laboriously making multiple measurements on many devices to learn what you need to know about device behavior, Kevin, thanks to his [machine learning] algorithm, can now tell you everything you'd want to know about a device and its properties from a single measurement that takes about 30 seconds."
The other novel feature of Lee's system is that it takes spatial maps of data from solar cells and converts them into images.
"Normally one of the most common measurements you get after creating a new solar cell is called a JV curve, and what it does is measure the cell's response to light," Lee said. "We had the idea of converting these JV curve maps into images so we could take advantage of advanced machine learning models developed for applications not in materials science, but in computer vision, to learn patterns in solar cell behavior."
Another benefit of the new method is its applicability to various materials and devices beyond solar cells, potentially accelerating the timeline from material discovery to market adoption.
"In theory, the system we developed could be used to measure other devices, such as transistors and light sensors," Lee said. "The time saved, and the accuracy of this system could lead to a wide array of new technologies being created much more quickly, which I am excited to see happen."