This article originally appeared in the Fall 2024 issue of Arts & Sciences Magazine
Artificial intelligence has recently exploded into the public consciousness, with chatbots such as ChatGPT becoming household words and Big Tech pouring hundreds of billions of dollars into AI's promise. At university schools of arts and sciences, including the Krieger School, the impact of AI is likewise being keenly felt across research, scholarship, and teaching.
While many major advances in AI as a technology are happening at the industry level, innovative applications are also springing up at universities. Alan Yuille, whose research at Johns Hopkins focuses on artificial computer vision systems, thinks this blossoming follows organically from the open, enterprising spirit of academia.
"There are certain things that companies can do that we can't because they've got lots more computer power and lots more data," says Yuille, a Bloomberg Distinguished Professor of computer science and cognitive science at both the Krieger School and the Whiting School of Engineering. "On the other hand, at universities, we're free to sort of do our own things and to explore different sets of ideas."
Particularly in the arts and sciences arena, where there is less of a short-term, profit-driven motive and more of a humanistic longer view, Yuille sees the benefits of broadly exploring AI in a university setting. Such an expansive approach may help shape the continuing evolution of the technology.
"In arts and sciences," Yuille observes, "it may be that you want to consider the good, long-term research, rather than research that you'll be able to put on a smartphone in a few months."
Here are several examples of how AI is opening new avenues of discovery and insight at the Krieger School.
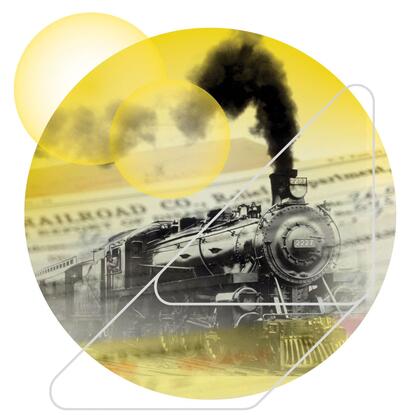
Image credit: STEPHANIE DALTON COWAN
Bringing the past alive
Known nowadays as a property in the board game Monopoly, the Baltimore and Ohio (B&O) Railroad was the country's first commercial railroad that operated for more than 150 years, from 1830 to 1987. Luckily for historians, the B&O Railroad Museum in Baltimore has preserved a treasure trove of insurance records the company kept for its thousands of employees, dating back to the early 1900s. Yet these records—a hodgepodge of about 16 million handwritten and typed pages in total—have sat in boxes for decades because human archivists could never plausibly catalogue them in any reasonable amount of time.
Recognizing the scale of the challenge, the B&O Railroad Museum consulted with Johns Hopkins professors and researchers. News of the massive data repository reached Louis Hyman, the Dorothy Ross Professor of History. A labor historian, Hyman teaches a course, AI and Data Methods in History, which explores the use of AI technologies as tools for research.
Based on this expertise, Hyman and other Johns Hopkins researchers are now working with B&O Railroad Museum curators on a project to scan and digitize the vast database. The project is employing optical character recognition, a kind of computer vision, to read letters in the documents. Researchers then run these texts through large language models, which correct for inevitable computer errors, but more importantly extract and organize the information in a way that allows researchers to search and aggregate the data.
"It's a one-of-a-kind database," says Anna Kresmer, the archivist at the B&O Railroad Museum, "and until now, until AI, there wasn't a practical way to deal with this data."
Creating research opportunities
Once made accessible, the B&O database should open up a plethora of research opportunities in labor, business, and medical history. Jonathan Goldman, chief curator of the B&O Railroad Museum, expects that with statistics-based approaches, "general trends will emerge from enterprising analysis of the data, for instance about certain types of injuries or how certain groups were treated."
"These records tell the lives of thousands of people," adds Hyman. "You can start to find patterns and excavate truths about the past that maybe we couldn't see before."
In coming years, Goldman says there is a vision to make the digitized documents accessible in public workstations at the museum, enabling visitors to conduct genealogical searches of relatives who worked for the railroad. "We'll help some families learn more about their ancestors, a bit about who they were, and what they experienced," says Goldman.
The project historians see AI methods becoming standard for tackling other, similarly large collections of written material. "AI has an incredible tolerance for boredom that no human has," Hyman says. "Using AI clarifies what is valuable about being human [when conducting research], and that's not sitting down and transcribing thousands of pieces of paper—it's thinking carefully and critically."
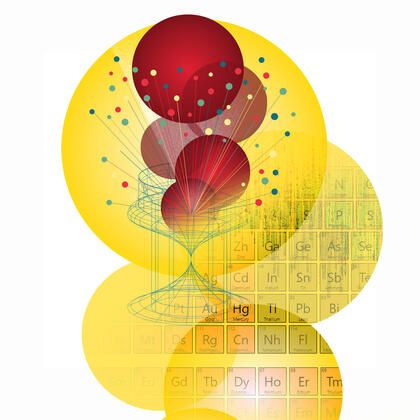
Image credit: STEPHANIE DALTON COWAN
Creating uncharted materials science
The lab of Tyrel M. McQueen is dedicated to synthesizing new materials to benefit society and provide insight into fundamental science. In this endeavor, artificial intelligence is emerging as a useful tool to drive discovery.
For instance, Queen was co-principal investigator on a project called MITHRIL—a clever acronym for Material Invention Through Hypothesis-unbiased, Real-time, Interdisciplinary Learning, named after a fictional metal in The Lord of the Rings. The project, along with follow-on research derived from it, has leveraged AI to rapidly explore vast possibilities of material combinations. For human researchers, canvassing millions of molecular configurations is impractically time-consuming, as well as limited in scope, because researchers sensibly work off what is already known rather than diving into random, undescribed space.
In collaboration with the Johns Hopkins Applied Physics Laboratory, McQueen and Krieger School colleagues recently engaged MITHRIL in a far-reaching search for new superconductors. When cooled to extremely low temperatures, these special materials conduct electricity without any loss of energy. While superconductors have numerous applications today, for instance in maglev trains and MRI machines, the Holy Grail for decades has been to find high-temperature superconductors that could revolutionize electrical power transmission, power storage, and other areas. "We'd love to find superconductors that don't have to be cool to work," says McQueen, a professor in the departments of Chemistry and Physics and Astronomy.
Algorithms for advances
The researchers provided MITHRIL with data on a couple hundred known superconducting substances, training its machine learning algorithms to make predictions on whether other materials would display the desirable property. The model performed admirably, "rediscovering" four described superconductors and revealing a fifth novel one. "Very quickly, we found things that are known superconductors but were not included in the training data set," says McQueen. "Then we also found a new superconductor that has never been made before."
The scientists proceeded to fabricate and test this new, AI-proposed superconductor, an alloy of zirconium, indium, and nickel that begins behaving like a superconductor at about 9 Kelvin, or -443° Fahrenheit. Though not a gamechanger at that frigidness level, the discovery nonetheless demonstrated AI's ability to bring about advances in materials science research.
Relatedly, McQueen is also using AI to better identify truly novel material ideas to improve the odds of identifying a previously unimagined substance that proves groundbreaking. AI models typically give two outputs—what the model thinks the right answer is, and how confident the model is in that answer being right. McQueen says the best kinds of answers are those where the model is most uncertain about a material's particular properties.
"That's the material you go and make, because that's the region of chemical space that hasn't been explored for that property before," McQueen says. "You use these methods to basically provide much better insight than any human can."
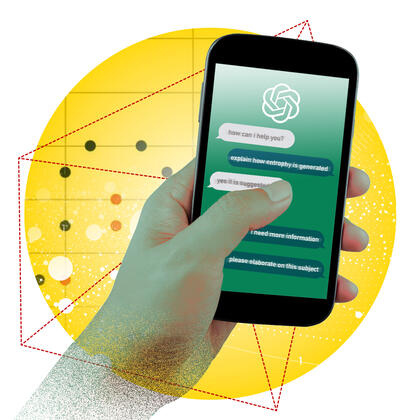
Image credit: STEPHANIE DALTON COWAN
Teaching with AI
One of AI's biggest impacts is in higher education, where the student use of chatbots remains hotly debated. Many fear that chatbots will fuel rampant cheating alongside bad scholarship, courtesy of chatbots' proclivity for arriving at bogus answers. Yet others hope these AI tools will serve as the latest example of an information technology that, when properly wielded, enhances learning.
To gauge AI best practices in student learning, sociologists at the Krieger School are avidly studying the patterns of how college students interact with chatbots. "AI requires a new type of information literacy," says Mike Reese, director of the Krieger School's Center for Teaching Excellence and Innovation and an associate teaching professor in the Department of Sociology. "It's important that we understand how students are adopting these tools."
Analyzing student-AI interaction
To this end, Lingxin Hao, a professor of public policy in the Department of Sociology, along with Reese and colleagues from the computer science department in the School of Engineering, is conducting field-leading research on campus with undergraduates. One key study took place over three weeks last fall. The researchers conducted an experiment with students in PILOT, a peer-led tutoring program where groups of roughly six to 10 students meet weekly to work on problem sets together.
First, the researchers created coursework-related questions via a Johns Hopkins-software-based quiz tool for the students to answer. Some student participants were randomly assigned to have access to ChatGPT, the popular online chatbot, and were further divided into groups that could either ask ChatGPT one question or ask multiple questions across rounds of interaction. The student prompts given to the AI and the responses received were all recorded in detail, so the total interaction could be parsed. "We got to see how humans and ChatGPT really interact," says Hao.
Overall, nearly 700 students took part, giving the researchers a rich dataset to analyze student behavior and performance with ChatGPT. Encouragingly, the researchers saw some students—especially those who reported familiarity with chatbots—demonstrate adeptness at using the tools as assistants; not simply as resources for leaping to potentially wrong answers, "but as guides for how to solve a problem and learn," says Hao. "The goal is to understand the social science foundation of human-AI interaction using the collected student-ChatGPT conversations."
Developing guidelines
The researchers are building on these and other findings in developing guidelines for teaching with AI. The study can help instructors integrate the burgeoning technology effectively and equitably into their classrooms. Doing so can not only advance learning objectives, but also equip students with a knowledge base for their future careers.
"It's critical for us as faculty to figure out how AI tools are going to be used in our discipline, whether it's in academia or out in industry, and then bring them into our classroom so students can be better prepared for using AI in their future careers," Reese says.
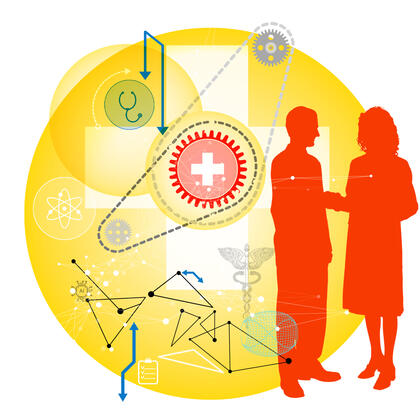
Image credit: STEPHANIE DALTON COWAN
Enhancing social care
For more than 20 years, through her studies in low-income urban neighborhoods in Chile, Clara Han has seen firsthand the challenges in providing social care services to vulnerable people, especially children. Social service workers often have insufficient resources and time to dedicate to each case—a problem amplified by a lack of data sharing between institutional entities such as health systems and welfare agencies.
"Frontline workers are constantly having to make decisions in terms of resource allocation and prioritizing one case over another, but there just isn't enough to go around," says Han, a professor in the Department of Anthropology.
As a result, care coordination is often suboptimal, and people in need can slip through the cracks, with tragic consequences. Han offers an example of a child with a medical condition who enters foster care without the agency informing her doctor. Because her newly assigned social worker cannot access her existing health records, the child ends up missing doses of a critical medication.
Han is interested in both regulatory environments that condition access to data as well as the impact of existing use of predictive algorithms in social services. To this end, along with pediatricians at Johns Hopkins University School of Medicine and colleagues across the university, Han is developing a research framework to look at the ethical and legal stakes in AI-powered decision support systems for medical care coordination in Baltimore City.
Testing AI's limits
Such decision support systems have become increasingly common in immigration and criminal justice settings. It is now clear that predictive algorithms can harm decision-making processes by exponentially "scaling-up" bias in social services. "You have what's called 'analog harm,' where people languish in limbo," Han describes.
Understanding the regulatory environment is crucial in order to improve data integration from diverse sources, Han says. At the same time, there is an increasing recognition of the limits of metrics when contending with complex social contexts. "AI can generate harms, just as any decision can generate harm," says Han. Thus, Han is interested in exploring how AI components, as part of decision-making processes, contend with the legitimacy of multiple stakeholders' claims. Even if these claims are contradictory. Given these multiple claims, any decision-making model for resource allocation must include a provision for human appeal.
Overall, Han sees tremendous promise for interdisciplinary research on AI, such that the expertise of frontline providers as well as their patients are actively brought into possibilities offered by algorithmic decision-making. "We want to move forward with our research framework," Han says, "because it could offer clear benefits for people right in Baltimore and other places with different technological digital milieu, where data-sharing laws might be quite different."
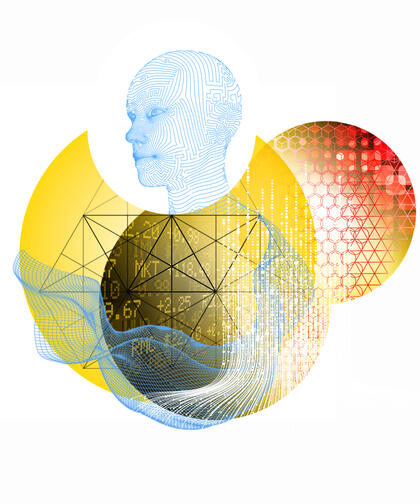
Image credit: STEPHANIE DALTON COWAN
Past is prologue
Henry Farrell is looking to get a handle on AI's potential impacts on the international world order, particularly as large language models (LLMs) become ingrained in society.
Farrell, the SNF Agora Institute Professor of International Affairs at the School of Advanced International Studies (SAIS), recalls his startling first encounter with a crude, early version of an LLM several years ago. As the model ran a Dungeons & Dragons-style online roleplaying game, it at times lost track of the user's character and made strange, even inappropriate responses and claims. Yet the technology's innate power and novelty still struck Farrell. "It was clearly very, very flawed," says Farrell, "but it was also clearly something that was genuinely new in the world."
Processing social information
Farrell has since studied LLMs within his purview of technology and political science. With LLM performance having dramatically improved over the past couple years, hand-in-hand with widespread adoption, Farrell and his colleagues are now examining how LLMs and machine learning more broadly function as a means of social information processing.
This ongoing analysis is informed by comparison with two historical, massively transformative social technologies: the market system and bureaucracies. Both systems, vast in scope and complexity, absorb gargantuan amounts of information—akin to the often-Internet-wide training sets of LLMs.
For the market system, all its devoured information is boiled down into supply-and-demand-driven prices for goods and services, while a bureaucracy condenses the aggregate of societal activity into categories for governmental oversight. LLMs perform a similar trick by poring over human knowledge corpuses to offer summarily brief responses to user queries.
In their enormity, all three technologies are far beyond anybody's comprehension and control, which breeds both terror and awe, Farrell says. "Many of the fears and the hopes that are associated with AI really are very similar to the fears and hopes that we have had about markets and about bureaucracies," says Farrell. Some of those fears have manifested, for instance in massive job losses as whole industries relocate or become obsolete due to market forces, or when government agencies ill-serve constituents. But many hopes have been borne out as well, from widely affordable food and consumer goods to successful governmental programs.
Thus, for insights into AI's ramifications now and in the future, Farrell and his colleagues are drawing lessons from the past. "We can learn to some degree from looking at these previous social technologies what kinds of shocks they inflicted," Farrell says. "That at least allows us to begin to ask the right questions, and that may give us a better sense of what's to come."
Posted in Science+Technology
Tagged artificial intelligence, humanities