For many diseases and chronic conditions, an individual's genes play a role in their likelihood of developing the disease. While some inherited diseases, such as cystic fibrosis or sickle cell anemia, are caused by a variation in a single gene, others, known as complex or polygenic diseases—such as cancer, type 2 diabetes, or heart disease—occur as a result of a combination of many gene variations as well as environmental factors.
Polygenic risk scores, or PRS, reflect a person's genetic susceptibility to a particular disease—a higher risk score expresses that an individual is more likely to get a disease. One significant limitation to date, however, is that existing risk scores have been developed and evaluated using primarily data from individuals of European ancestry. The resulting risk scores, and the algorithms for calculating them, are not as accurate for understudied minority populations, especially in populations of African ancestry.
To address this issue, researchers at Johns Hopkins University in collaboration with the Harvard School of Public Health and the National Cancer Institute, have developed a new method with significant potential to improve the performance of polygenic risk scores in minority populations. The method, called CT-SLEB, is detailed in a paper published in Nature Genetics.
The study was supervised by Nilanjan Chatterjee, Bloomberg Distinguished Professor of biostatistics and genetic epidemiology at Johns Hopkins, who says it's crucial to have both better methods and more data from diverse populations.
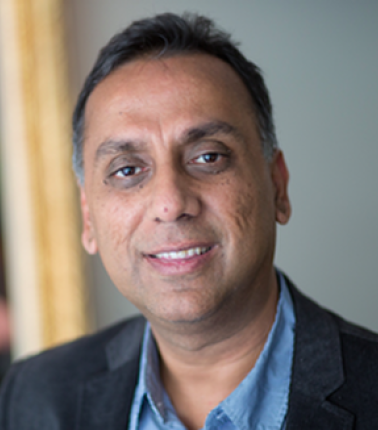
Image caption: Nilanjan Chatterjee
"Better methods are important, but those alone will not eliminate the performance gap of these models across diverse populations," Chatterjee says. "We also need to collect more data on various minority populations so that these models can be better trained and the accuracy of genetic scores for these populations is improved. In terms of disease risk, most of the underlying biology is the same, so the same genetic variants in the same region of the genome will most likely have a similar effect on disease risk across populations. There may, however, be genetic variants unique to individual populations, so it is essential to have this information included in calculations in order to have the most accurate risk predictions for all populations."
The CT-SLEB method combines aspects of several existing techniques, including the clumping and thresholding (CT) method, an empirical-Bayes (EB) approach, and a super-learning (SL) model. In total, the researchers analyzed more than 19 million genetic variants from data on more than 5 million people, including many from understudied minority populations. When the researchers evaluated CT-SLEB using large-scale genome-wide association studies across diverse ancestry populations, CT-SLEB showed promising results in terms of performance and scalability for future use.
Genetic risk scores have emerged as a promising tool for identifying individuals who might benefit from interventions because of a high risk of a specific disease. Yet, the inequality in performance of polygenic risk scores across populations raises concerns that using this technology in clinical settings may further exacerbate health care inequities.
"There has been a lot of excitement about the possibility of moving information from polygenic risk scores from research into clinical practice—the potential value of using genetic risk scores for targeting interventions is significant," Chatterjee says. "The problem is the genetic scores that have been derived so far don't perform as well in African ancestry populations. As we know, there are already a lot of barriers that lead to health care disparities, and we don't want to introduce another. We need to push toward collecting more data and developing better algorithms to allow for an equitable approach to bringing this new technology into the field in a way that is inclusive and benefits everybody."
The study's lead author, Haoyu Zhang, who began the project as a doctoral student in the Johns Hopkins Bloomberg School of Public Health and is now an investigator at the National Cancer Institute, says these findings have broad implications for improving health equity by enhancing polygenic risk predictions across diverse populations.
"Better PRS performance can lead to more accurate risk prediction, which facilitates early disease detection, prevention, and personalized treatment strategies," Zhang says. "Our novel CT-SLEB method, along with other emerging methods, enables the unique genetic architectures of underrepresented populations in genetic research to be considered and might therefore contribute to reducing health disparities."
Chatterjee says that the potential inequities through clinical application due to unequal representation in genetic studies exemplifies a major concern about the broader impacts of racial bias in algorithms, including those developed by artificial intelligence methods, and why it is essential to look for these biases as early as possible in order to minimize damage.
"If not properly implemented, AI can introduce very systematic biases into society," Chatterjee says. "Many existing clinical algorithms and technologies that have very serious implications for health care outcomes have been developed and evaluated for people of European origin, which people are now questioning. With AI and machine learning algorithms, you have to be really careful about these biases being introduced early on, because once they become deeply entrenched into the system, it is difficult to change practice. I think it is crucial for us to be aware of and talk about these biases to try to address the existing and prevent future ones."
Added Elizabeth Stuart, chair of the Department of Biostatistics at the Bloomberg School: "This work highlights the importance of paying attention to where data comes from, who is represented, and, crucially, who is not represented, in traditional data sources. Through strong multidisciplinary collaborations of individuals who understand the substantive and technical aspects, creative use of new data sources, and the development of statistical methods to handle complex data we can make important advances in improving health for individuals across the world."
In addition to improved performance for diverse populations, CT-SLEB has a quick runtime, which gives it the capacity to analyze data and calculate polygenic risk scores much faster than other methods. CT-SLEB is able to computationally adapt quickly to increasingly complex settings, which makes the method scalable to larger data sets in the future, as it can easily handle larger numbers of genetic variants and additional populations. The software code for the method and all analyses have been made publicly available through a GitHub repository. The team is further developing additional methods that are also computationally fast and yet can further enhance the accuracy of risk scores through more advanced modeling.
"Some of the existing machine learning methods can be computationally very daunting, especially to analyze such enormous amounts of data," Chatterjee says. "We took ideas from machine learning and Bayesian modeling literature, but implemented them in such a way to create a computationally simple and powerful method that can analyze data on a massive scale and do the required calculations in a reasonable amount of time. This will hopefully allow us to analyze more data on additional populations in the future to improve polygenic risk scores."
Chatterjee says that much of this project's success comes from the level of collaboration involved, which enabled the team to leverage knowledge and resources across academia, government, and industry, including a partnership with the company 23andMe. 23andMe provides genetic testing to individual customers, who send in saliva samples for analysis and receive a report on their ancestry, genetic predispositions to health-related topics, and other relatives who have also submitted their DNA.
To protect the privacy of individuals who submit their DNA to 23andMe, the company does not release individual level data. The study required a coordinated system between researchers at Johns Hopkins, Harvard School of Public Health, and contacts at 23andMe in order to test various risk calculation methods by sending a series of analyses and results back and forth. This collaboration was based purely on research—the authors of the study who are not 23andMe employees do not have a financial relationship with the company.
"The collaboration with 23andMe was very unique for this project," Chatterjee says. "This partnership gave us access to unprecedented large and diverse datasets to evaluate and compare a variety of polygenic risk score methodologies, including CT-SLEB. I don't think there's any other paper that has been able to analyze this volume of data before, and that was only possible because of the collaboration with 23andMe."
Posted in Health
Tagged genetics, health disparities, machine learning, nilanjan chatterjee