Ask biostatistician Scott Zeger about the revolutionary changes he sees on the horizon for medicine, and the first thing he does is rewind to the 1600s. Medicine then was mostly a primitive matter of luck and guesswork. Every doctor had his own theories about what worked and why, and none of those theories was based on anything we would call science. "What they did back then was closer to the barbering profession than what we think of today as medicine," Zeger says. Then came the microscope. As the 17th and 18th centuries progressed, scientists in the fields of microbiology, immunology, and other emerging fields could observe biological processes in greater and greater detail. For the first time, they could develop and disseminate observation-derived knowledge about the inner workings of the human body. Discoveries piled one atop another—red blood cells, spermatozoa, microorganisms, and many more. In Zeger's telling, these discoveries gave birth to modern medicine. By the mid-1800s, doctoring had left its barbering days behind and become a recognizably modern endeavor. What had been all luck and guesswork now was built on a foundation of biological science.
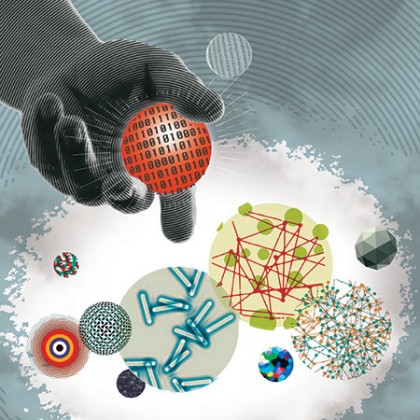
Image credit: ANDY MARTIN
What Zeger, who served as Johns Hopkins' vice provost for research from 2008 to 2014, sees on the horizon today are onrushing changes that will add up to a second revolution as dramatic and encompassing as the first. "What we're talking about here is the transformation of medicine," he says. "The biomedical sciences have been the pillar of the health care system for a long time now. The new system will have two equal pillars—the biomedical sciences and the data sciences."
By the latter, Zeger means "big data." Big data, it seems, is going to be a big deal. Especially at Hopkins, where Zeger directs the Johns Hopkins Individualized Health Initiative, meant to ensure that the institution plays a major role in this coming revolution, if that is what it proves to be. Known in institutional (and typographical) shorthand as inHealth, the effort is one of five signature initiatives in the seven-year, $4.5 billion Rising to the Challenge capital campaign that began in 2010. In a May 2013 news release, the university described inHealth: "Physicians, scientists, engineers, and information experts will help doctors customize treatment for each patient by connecting and analyzing huge databases of clinical information, plus new data sources such as DNA sequences, methylation analyses, RNA expression levels, protein structures, and high-tech images." A survey of its website reveals inHealth participants from Johns Hopkins Medicine; the Hopkins schools of Medicine, Public Health, Engineering, Arts and Sciences, and Nursing; Carey Business School; the Berman Institute of Bioethics; the Applied Physics Laboratory; and Johns Hopkins HealthCare.
Hopkins researchers have already launched multidisciplinary inHealth pilot projects to test concepts in several areas of medicine. Zeger is not much interested in running an enterprise that contributes mostly theories and predictions. "You can talk about big ideas until you're blue in the face and not really get anywhere," he says. "This initiative is all about us getting out there and doing these demo projects. Then we'll be able to point to actual results and say, 'See, this is the sort of thing that can happen when the data sciences and the biomedical sciences work more closely together.'"
To qualify as "big" in the sense that information scientists use the term, a dataset must reach a level of size and complexity that it becomes a challenge to store, process, and analyze by standard computational methods. This threshold changes constantly. Researchers Martin Hilbert and Priscila López estimated in 2011 that per capita computing capacity has been doubling every 40 months since the 1980s. The first time a human genome was decoded, processing the 3 billion "letters" of genetic code took a decade. That was in 2000. Fifteen years later, a human genome can be decoded in less than a day. Some of today's big datasets will no longer qualify as big a few years down the road. However one defines it, big data has been slow to arrive in medicine. One problem has been a lack of functional data. Clunky, old-fashioned ways of keeping records have hung around as standard practice. Paper charts have survived into the 21st century. When records have made it onto computers, they've often done so in formats that information sciences cannot do much with—audio dictation of patient exam notes or lab results stored as PDFs made from barely legible faxes, for example.
Three things have catalyzed the embrace of big data by medicine. First was the 2009 American Reinvestment and Recovery Act, which created financial incentives for physicians to adopt electronic health records. By mid-2014, 75 percent of eligible doctors and 92 percent of eligible hospitals had signed on for the incentives. Second, complex new sources of data, such as the aforementioned protein structures and RNA expression levels, along with an array of advanced imaging technologies, have come into play. To use them requires sophisticated new biotechnology capabilities. Finally, there is the changing nature of health care as a business. To keep costs down and improve patient outcomes, health care reformers are looking to transform the business model of medicine into one where the bottom line is based not on volume of services but on efficiency and health outcomes. Looking ahead, Zeger says, this change will place a premium in medical practice on the type of intensive, outcomes-oriented database mining that has been so successful in the world of online commerce, where the data-tracking practices of Amazon and Google are the most-cited examples.
"There are going to be new financial realities in our health care system," Zeger says. "The days when we all could get by without being 'Google-esque' in our analytics are just about gone."
Back in 1991, a prescient Johns Hopkins rheumatologist named Fred Wigley decided to play a little long ball. As he and co-founder Robert Wise developed a vision for the fledgling Johns Hopkins Scleroderma Center, they found themselves pondering the uselessness of so much data kept on this rare autoimmune disease. "Different doctors might have 20 patients here or 50 patients there or 100 patients somewhere else," Wigley recalls. Different doctors were "collecting their own individual kinds of data, and they were storing it in their own individual ways." Almost no data were being collected with prospective and longitudinal approaches, he adds.One of the many ways scleroderma can make life miserable is by a painful tightening of the skin. In the early 1990s, one physician might be tracking that phenomenon by its location in the body—is the tightening in the fingers, the forearms, or both? Another physician might focus not on location but on severity, with no record of where the tightness occurred. Wigley and Wise decided to take a different approach at their new center. They set a protocol in which the same clinical measurements would be taken and the same biological samples collected from every patient. They wanted the Scleroderma Center to pioneer the development of a useful longitudinal database that would allow them to make the most of new technologies and discoveries as they arrived in the years to come. Wigley expected that, at a minimum, it would help future researchers take retrospective looks through the data in search of the natural course and distinct patterns of the disease. "At a place like Hopkins, you're always looking at the history of medicine and seeing discoveries and how things are always changing," Wigley says. "When we started collecting this information, we definitely had the idea that in the future we'd probably run into some new things to do with it."
Twenty-four years later, Wigley's longitudinal database encompasses more than 3,200 patients and 18,000 individual visits to the center. "For a rare disease like this—it only affects about 300,000 people in the country—those are big numbers," says Laura Hummers, a rheumatologist and co-director of the center. "That's really what put us in a position to be a part of inHealth—we have this rare, complicated disease, but we have really good longitudinal data. That's the sort of situation where maybe we can create a model for how this might work in other rare diseases."
The database is at the heart of an inHealth pilot program that recently won National Science Foundation backing to the tune of four years and $1.4 million. The goal of the project (branded as inADM for "individualized autoimmune disease management") is to find in that database a better understanding of which patients are headed along which of scleroderma's trajectories. Autoimmune diseases tend to be unpredictable disorders that can take a variety of different trajectories, from mild and slow to devastating and fast. Scleroderma is just such an illness, presenting differently across different patients. Some develop pulmonary hypertension; others don't. Some show active skin disease throughout; others don't. It can cause trouble with a patient's esophagus, blood vessels, kidneys, and bowels. But there is generally no way to predict which troubles will arise in which cases and how serious they will be. "It can be hard for a physician, even one who sees as many cases as we do here, to decide how to proceed with any individual patient," Hummers says.
Consider just one effect: decline of lung function. For most scleroderma patients, pulmonary function is relatively stable and becomes an issue only during significant physical exertion. But one in 10 patients suffers from a rapid buildup of scar tissue in the lungs, causing intense shortness of breath that makes the simplest of day-to-day activities a challenge. "We have medicines that can help in preventing the progression of lung decline," Hummers says. "But those medicines are toxic and we don't want to give them to everyone, just to the people who need them. But right now what we have are lots of people who start out looking the same. We can't pick out the ones who are headed for rapid lung decline until it happens."
Suchi Saria, an assistant professor of computer science in the Whiting School who also holds an appointment in health policy at the Bloomberg School, will be looking to solve that conundrum—and others like it—once she loads all of the Wigley data, cleans it up as best she can, and commences trying to find ways to use it for more reliable predictions. She is collaborating with Wigley and other investigators at the Scleroderma Center to study how to identify scleroderma subtypes or homogeneous patient subgroups. Then, they will study whether we can predict an individual's disease course as early as possible by inferring their subtype. "Our early results have uncovered that there is not one but many different subtypes," she says. "When looking at lung function alone, we see that some patients stay stable, others decline throughout their course, and yet others show rapid early decline but stabilize. We also see some patients who are stable for the most part but then surprisingly show rapid decline late in their course. These patterns allow us to ask why different individuals show these different disease trajectories."
Saria notes that working to develop models means "doing a deep dive to really understand all the ways in which the data are messy. To build robust models, we must deeply understand the data and how they were collected. If you aren't careful with data like this, it can be highly dangerous. It can lead you to conclusions that just aren't right." Her team has realized they need to carefully understand protocols that guide what data get recorded, and the ways in which errors can occur. "For example, we noticed from our analysis that many patients seemed to not be receiving adequate therapeutic interventions prior to 2003. Well, turns out, they'd switched to a new computerized physician order entry system in 2003, so medication data prior to that were recorded in paper charts and only selectively uploaded to the database." More danger spots: Because of improvements in technology, are data from 1997 less precise or reliable than comparable data from 2014? Can a predictive model account for the possibility that some patients made six clinical visits over 10 years while others came in 40 times over six years? What about the fact that different patients received different treatments over the course of their visits? Will it be possible to unconfound those treatment effects and drill down to the point where researchers are confident they can see what the innate course of the disease would have been in the absence of treatment? "There are some things we are looking at here where we will need to develop new methods," Saria says. "We just don't have some of the tools to do some of these things now. There is a gap in terms of current computational and statistical approaches and what is needed to fully embrace the messiness of electronic health records data. We think we're at a stage where we can really make progress on these goals, and in our scleroderma work we're already starting to see early promising results."
As useful as new predictive tools might be for physicians at the Johns Hopkins Scleroderma Center, they could be even more beneficial in the field, where scleroderma patients are rare and physicians never have a chance to gain significant experience in treating them. Says Zeger, "What we're looking to do is take that special brand of experience and intuition that the best doctors have and turn it into something that has a scientific foundation based in data and can eventually form useful, effective practice tools that we can put in the hands of clinicians here and around the country and the world."
In 2013, the American Urological Association revised its guidelines for prostate cancer screening, taking aim at two problems associated with prostate-specific antigen tests. One is false positives that put families through unnecessary cancer scares. The other is a phenomenon doctors call overdiagnosis. Counterintuitive as it might seem amid so much emphasis on early detection across all types of cancer, there are times when people would be better off not knowing. Between 20 and 50 percent of the tumors diagnosed under the old prostate-specific antigen test guidelines would never have grown big enough to be a problem during a patient's lifetime. But at this point, doctors are unable to predict which tumors will turn out to be slow-growing—and the result is a good number of men are receiving treatments with significant side effects for tumors that would never have caused problems. Previously, the American Urological Association recommended that prostate-specific antigen testing be a routine part of annual physicals for men over age 40. The new guidelines call for no testing for men younger than 55 or older than 70 and at a normal level of risk. For those at normal risk in between those ages, prostate-specific antigen testing is an option for patients to consider, but not one that comes recommended as routine. For those who decide to get tested, the American Urological Association also recommends a two-year interval between tests, as opposed to the previous one-year interval.These changes caused quite a bit of confusion in the primary care community, and that confusion soon drew the attention of the inHealth team working to develop pilot projects that aim to optimize cancer screening for the 275,000 people covered by insurance through Johns Hopkins. (Their project has been dubbed inCAS—individualized cancer screening.) They began looking at whether and how experts at the Kimmel Cancer Center might reach out to the network of Hopkins primary care doctors and help with prostate-cancer screening decisions—and, perhaps, screening decisions for other cancers as well.
To understand the scope of the prostate confusion, cancer researcher Craig Pollack surveyed providers in the Johns Hopkins Community Physicians network. Responses to the new screening recommendations ranged from, "It's about time," to, "How dare they!" to, "Yay on this but nay on that." A good number of doctors basically said they planned to ignore the new guidelines. "The results were eye-opening," says Johns Hopkins epidemiologist Elizabeth Platz. "The resistance to guidelines that were based on a review of all the available evidence—essentially, some of these doctors seemed to be saying that they don't believe the research."
Platz leads the inCAS team, which includes epidemiologists, biostatisticians and other cancer researchers, practicing physicians, and health care executives. In surveying the landscape ahead as the initiative got off the ground, they soon realized that they were at square one in the effort to bring big data to bear on cancer-screening decisions. Reliable data weren't available to answer some rather fundamental questions: How many Hopkins-insured persons follow current recommendations for cancer screening? How many are getting screened at all? Which cancers are they getting screened for, and at what intervals?
The inCAS team is working toward a day when longitudinal data exists on every cancer-screening step taken in those quarter of a million Hopkins-insured lives, from the first screening straight through to whether patients ever receive a cancer diagnosis and, if so, their outcomes. They are looking not just at prostate cancer but at colorectal, breast, lung, and cervical cancers as well. "There is a need to do better, and that need is all across the spectrum of cancers," says Johns Hopkins urologist H. Ballentine Carter. "But until we know where we are, it's going to be hard to know where to go next."
A second survey of Johns Hopkins Community Physicians is underway, this one for colon cancer screenings. In addition, inCAS researchers are working with Johns Hopkins Urban Health Institute researchers as they conduct a series of focus groups with people in East Baltimore who should have had a colonoscopy by now but have not. What's stopping them? Is it fear of the procedure? Is it logistical problems, like not having access to a ride home afterward? Is it more complicated, like being homeless and having nowhere to do the bowel-emptying prep work? (One focus group is specifically reserved for the homeless.) "We need to figure out what we're doing in cancer screening and then we need to think about how to do it better and more consistently," Platz says.
Looking ahead, Platz says the inCAS team hopes to develop new clinical tools that help physicians and patients make cancer-screening decisions. Pollack recently submitted a grant application with the nonprofit Patient Centered Outcomes Research Institute that would allow him to work with software experts on the design of a preliminary prototype for just such a practice aid.
Over at the Brady Urological Institute, Carter is looking forward to the day when all this work of constructing a big data infrastructure in cancer screening is complete. He is hopeful that the database will hold the key that helps solve the problem of overdiagnosis in prostate cancer once and for all. "I think that this data-driven approach that is constantly looking at outcomes will really help us, over time, understand which prostate cancer patients can be safely monitored and when patients need to be treated," Carter says. "This is where we should be headed, toward a process where we learn more about the population as a whole from studying individuals, and then we learn more about the individual from studying the populations. That's a process that can continuously renourish itself in terms of knowledge."
The Institute of Medicine began to publish papers a few years ago that argued the time had come for health care to embrace systems engineering to manage ever-increasing complexity and change. In 2012, Alan Ravitz took charge of a team from the Applied Physics Laboratory that collaborated with the Armstrong Institute for Patient Safety and Quality to take a systems approach to reducing medical errors in intensive care units. The Armstrong group defined the "as-is" state of patient safety in the ICU and worked with the APL team to develop concepts for a "to-be" ICU where medical errors would be reduced through the integration of technology and workflows. The team set about employing the hallmarks of systems engineering, an array of brainstorming, planning, and decision-making processes that have been used to help a number of other industries take steps forward in the areas of efficiency, safety, and quality. Ravitz, a health care program area manager at APL, soon realized the processes that were so familiar and time-tested to engineers came as a surprise to health care professionals. "On numerous occasions we heard comments such as, 'We normally do not get asked what we want our new systems to do, how they should function, and what they shouldn't do,'" he wrote in a 2013 essay about the project in APL Technical Digest.Nevertheless, says Zeger, "systems engineering is going to play a really key role in what we do with inHealth. These engineers are going to help us make sure that innovations end up producing the desired outcomes in the wards and in the labs. Too often in medicine, new things mainly succeed in just adding another layer of complexity to a workplace." APL engineers have begun work on inCAR, which aims to re-engineer the way things are done in the cardiac catheterization lab. The cath lab can be a pressure-packed place. Clinicians performing a catheterization guide a tube through an artery from a patient's groin to the heart, gauging their progress on a video screen that shows all the major blood vessels to the heart. At the same time, instruments at the tip of the catheter measure the blood pressure in each of the heart's chambers and connecting vessels, inspect the interior of the blood vessels, and take blood and tissue samples from various places inside the heart.
Systems engineer John Benson took part in initial meetings last summer between the APL team and some cardiac clinicians. "The first step is to work with clinicians to understand the problem space," Benson says. "How, exactly, are they doing things today? And how, exactly, do they want to be doing things tomorrow?" Benson points out that many cath lab decisions must be made under pressure during the procedure, and the data that doctors need to make good decisions can be spread out in different parts of the system, a complication that means they don't always have ready access to everything they need. What systems engineers do in such a case is unwrap a problem like that in great detail. What is the workflow in the lab? Who takes in which pieces of information and where do they put them? Can that system be improved, and if so, how?
"John and I have seen up close over the years how this approach has been a remarkable success in the aviation industry, in ground transportation, and in defense systems," Ravitz says. "The notion of constantly learning from real world experience in order to improve future performance is a routine thing now in those industries. We believe that there are going to be numerous opportunities to take these approaches and use them to improve outcomes and efficiencies in health care."
If there is one place at Johns Hopkins where big data medicine is close to up and running, it's radiation oncology. A pilot project there called Oncospace is on the way to demonstrating what inHealth advocates call a "learning health system." The brainchild of radiation oncology physicist Todd McNutt, Oncospace is a database of diagnostic 3-D images of tumors from thousands of past patients with head and neck, prostate, or pancreatic cancers. These images are matched to a wide range of data about those patients—anatomy, comorbidities, radiation dose distribution, treatment side effects, case outcomes, and more.Traditionally, a radiation oncologist studies images from a cancer and then fashions a plan of attack based on his or her experience with and memory of similar cases. With Oncospace, that's only the first step. The second step is tapping the database to test-drive a draft treatment plan. As described by Zeger, Oncospace allows oncologists to study documented outcomes of similar treatment plans with similar patients in the past. They can also explore the efficacy of potential alternatives. The database is more accurate and comprehensive than anyone's individual memory. Early tests in both head and neck and pancreatic cancers have found that using Oncospace boosts the effectiveness and safety of radiation regimens. "Todd's work on this is really one of the first demonstrations of how we can develop large data warehouses of patient information and use it to make individualized treatment decisions for new patients," says Theodore deWeese, chair of Radiation Oncology and Molecular Radiation Sciences at Johns Hopkins.
Zeger says the vision is for Oncospace to grow in exponential fashion. McNutt and his colleagues are now putting a new database together for lung cancer, a disease where radiation planning tends to be much more complicated than in other cancers. Zeger anticipates that Oncospace will eventually extend its reach to any cancer where radiation is commonly used in therapy. Growth will also come through the development of outside partnerships. Hopkins oncologists see only about 250 head-and-neck cancers a year, McNutt says, but the Oncospace initiative is now partnering with the University of Washington and the University of Toronto so that cases from those institutions are added to the database. The more patients in the system, the stronger the database will perform. Zeger says that he expects to see more of these partnerships take shape in the years ahead.
Amazon and Google learn about people's likes and habits from each new keystroke they make on a computer. So, too, physicians can learn from each treatment's outcome as recorded in a database. "That's a big principle in this project—the idea of embedding scientific inquiry into clinical care in ways that are systematized and powerful," Zeger says. "We want to be in a position where we are constantly capturing data about which decisions are producing the best outcomes for which subsets of patients."
The analogy Zeger and other big data proponents turn to most often in talking about the future is that of an air traffic controller. The idea is to picture each patient as a plane flying through the various ups and downs of life. The information sciences promise to give clinicians powerful new tools akin to the radar used by traffic controllers to see where any one plane is headed and then help that plane steer clear of traffic, turbulence, storms, and other dangers. There is a lot of talk about "health trajectories" in inHealth circles. Providers using big-data tools in the decades ahead may have better answers to questions that are often unanswerable today. Is a particular cancer dangerous, or will it likely be slow growing and thus safe to monitor without treatment? Is a newly diagnosed case of lupus one that will eventually present life-threatening kidney problems? Which is the best intervention for a specific cardiac patient? Carter says, "What we're trying to do here is learn more and more about where our patients are headed—their individual trajectories. We want to understand more clearly the risks they face and see, on that individual level, how and why one person's risks are different from another's."
In talking up inHealth, Zeger likes to point out that this notion of human disease as an individualized phenomenon is not a new concept. He makes regular use of a quote that dates back to the early days of academic medicine in this country. It's from a speech that Sir William Osler, the founding physician-in-chief of Johns Hopkins Hospital, gave to the New Haven Medical Association in 1903. "Variability is the law of life," Osler said. "As no two faces are the same, so no two bodies are alike, and no two individuals react alike and behave alike under the abnormal conditions which we know as disease." The big data future is all about turning Osler's observation into a scientific discipline—one capable of utilizing information sciences and putting powerful new tools in the hands of physicians.