Civil Engineering Seminar: Karen Willcox
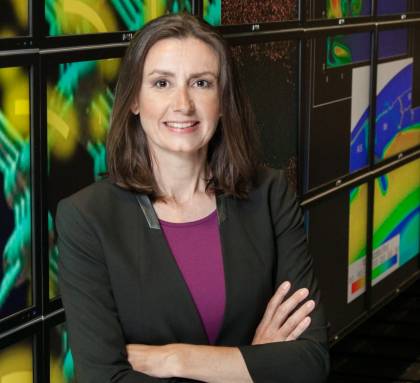
Description
The Johns Hopkins Department of Civil Engineering is pleased to welcome Karen Willcox, director of the Institute for Computational Engineering and Sciences at the University of Texas at Austin, for a seminar titled "Projection-based Model Reduction: Physics-based Approaches to Learn Low-dimensional Models."
Abstract:
The field of model reduction encompasses a broad range of methods that seek efficient low-dimensional representations of an underlying high-fidelity model. A large class of model reduction methods are projection-based; that is, they derive the low-dimensional approximation by projection of the original large-scale model onto a low-dimensional subspace. Model reduction has clear connections to machine learning. The difference in fields is perhaps largely one of history and perspective: model reduction methods have grown from the scientific computing community, with a focus on reducinghigh-dimensional models that arise from physics-based modeling, whereas machine learning has grown from the computer science community, with a focus on creating low-dimensional models from black-box data streams. Yet recent years have seen an increased blending of the two perspectives and a recognition of the associated opportunities. This talk will describe a model reduction approach that combines lifting-- the introduction of auxiliary variables to transform a general nonlinear model to a model with polynomial nonlinearities--with proper orthogonal decomposition. The result is a data-driven formulation to learn the low-dimensional model from high-fidelity simulation data, but a key aspect of the approach is that the lifted state-space in which the learning is achieved is derived using the problem physics. The method is demonstrated for nonlinear systems of partial differential equations arising in rocket combustion applications.
Who can attend?
- General public
- Faculty
- Staff
- Students