- Name
- Jill Rosen
- jrosen@jhu.edu
- Office phone
- 443-997-9906
- Cell phone
- 443-547-8805
Although the popularity of solar energy has surged, the unpredictability of a weather-dependent technology has kept even more people from embracing it. A new Johns Hopkins University-led project hopes to change that by improving our ability to forecast sunshine and backup power needs.
During the three-year effort, funded by a nearly $2 million grant from the U.S. Department of Energy's Solar Energy Technologies Office, the team will develop a new weather prediction system. Johns Hopkins engineers will then use that system to build a model that will more precisely estimate a region's solar and traditional power needs on any given day, saving potentially tens of millions of dollars and cutting energy waste.
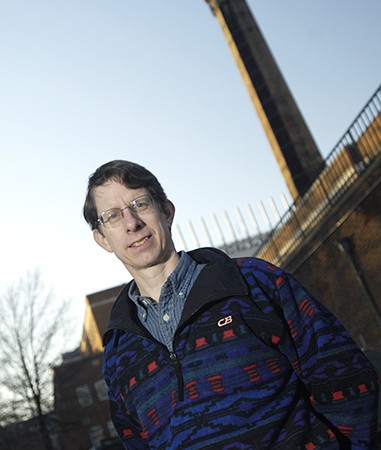
Image caption: Benjamin Hobbs
"This has the potential to do the economy, the environment, and consumers a lot of good," said project lead Benjamin F. Hobbs, a professor of environmental management in the Department of Environmental Health and Engineering at Johns Hopkins. "This is why I do research—we can help people with real problems."
Solar and wind power accounts for about 10 percent of all electricity produced in the U.S. With costs dropping for consumers, solar is expected to grow another 25 percent worldwide in the next year, Hobbs said. That's up from essentially no one using it in 1999.
The technology, of course, requires sunny days to generate power. When sunshine is in short supply, solar customers need traditional power sources or storage to tide them over with electricity. But there's little storage available in most places, and back-up power suppliers haven't been able to adequately prepare for this fluctuating demand, Hobbs said.
Currently, traditional power suppliers, who have to predict how much power a region will need a day in advance, over- or underestimate this demand by about 5 percent. The calculation will only become trickier as more people choose solar options. Those errors are not only costly, they can waste fuel and increase pollution if the guess is too high, and or risk power shortages if it's too low, said Hobbs, who also directs the university's Environment, Energy, Sustainability and Health Institute.
The goal of Hobbs' project, which started this month, is to significantly improve solar forecasting using artificial intelligence and data analytics so the nation's grid operators know precisely, and in advance, how much power to produce for any given day and hour. They will test the system initially in California and in the central part of the U.S.
Hobbs believes his team's plan for a smarter model will reduce errors by as much as 20 percent and, by eliminating that waste, will save major power grid operators tens of millions of dollars a year.
"The Department of Energy realizes if they can produce better solar forecasts, we'll get more cost savings and pollution reductions from the solar we already have," Hobbs said. "And then people will become even more willing to invest in renewable technologies."
Johns Hopkins will work with IBM's Thomas J. Watson Research Center, the National Renewable Energy Laboratory, the University of Texas at Dallas, and power grid operators in California and the central portion of the U.S.
Posted in Science+Technology
Tagged environmental engineering, solar power